Computational Anatomy
Research team
Computational Anatomy work closely with the SPM team, with the aim of incorporating many of their new algorithms into the SPM software. These contributions mostly relate to more accurate alignment among 3D scans, which involves both the relatively simple problem of within-subject alignment, as well as the more difficult problem of aligning the dissimilar shaped brains of different individuals together. For studies using fMRI scans, within-subject alignment helps to correct head motion within the scanner and align scans that may have been collected on different occasions, whereas between-subject alignment helps to ensure that the brain activity from different individuals is correctly superimposed.
A useful technique for clinical researchers is segmentation of brain scans into different tissue types, which allows regional volumes of brain structures to be compared among different populations of individuals. This sort of cross-sectional analysis has been widely applied by the brain imaging community through a technique known as “voxel-based morphometry” (VBM), which has the aim of localising where volumetric differences occur. This type of approach can be done using the SPM software. Another technique involves applying machine learning to maps of tissue types to make predictions about individuals, such as some diagnosis or outcome measure. The latter technique may be applied through a different software package that the group contribute towards (PRoNTo).
In collaboration with Parashkev Nachev’s team, some of the algorithms developed by the Computational Anatomy team are also being applied to patient scans within the National Hospital. This has motivated some work on super-resolution for recovering more useful information from collections of thick-sliced clinical scans. A simplified version of this algorithm has also been applied to denoising MRI scans. Relatively recently, this collaboration has led to us working with deep generative modelling approaches (a sort of “AI” for images) with the aim of synthesising high-dimensional, multi-modal clinical and neuroimaging data and developing new machine learning tools to enable accurate predictions that may inform clinical decision-making.
Many neurodegenerative disorders show characteristic patterns of brain atrophy. For example, atrophy in the brains of patients with Alzheimer’s disease is most clearly visible in those parts of the brain associated with memory. Another computational anatomy tool within SPM allows maps of regional shrinkage and expansion can be derived by nonlinear alignment of scans of the same individual taken a few months apart.
The Computational Anatomy team works closely with members of the Physics team. Earlier collaborations led to the “UNICORT” approach for estimating and correcting artifacts, which enables better quantitative measures from MRI. Now, we are finding that several of the techniques we previously developed can be applied to solving problems encountered when reconstructing quantitative maps from MRI data. This includes a correction approach, previously used as part of the tool for tracking brain atrophy, but which is now modified to help reconstruct multi-coil MRI scans.
Computational Anatomy work closely with the Anatomical Phenomics team, with the aims of generating imaging biomarkers for Parkinson’s disease, and also of increasing the accuracy with which certain difficult-to-see brain structures may be localised. In particular, the ventralis intermediate nucleus (Vim) is a small region within the thalamus that is a target that neurosurgeons aim for when using deep brain stimulation to alleviate tremor.
Team
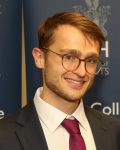
Guilherme Pombo
Fabio Ferreira
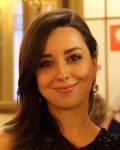