Threat Avoidance
Research team
We investigate how the neural system forecasts threat and selects preprogrammed survival actions, with the goal of developing novel psychiatric treatments.
Threat to survival comes in many forms: starvation, predation, self-defending animals, accidents, or inter-human conflict. The human brain has access to a large repertoire of defensive actions.
A requirement to act ultra-fast and with high precision poses interesting computational challenges. Emitting defensive actions in the absence of threat is a hallmark of several psychiatric conditions. By combining behavioural assays with neuroimaging and pharmacological intervention, we seek to understand how the neural system predicts threat and selects appropriate action. Novel interventions to rectify inappropriate threat prediction are tested in experimental models of psychiatric disorders.
Ongoing studies
ActionContraThreat
To elicit threat-related action in the laboratory, we use virtual reality computer games with full body motion, and track actions with motion-capture technology. Based on a cognitive-computational framework, we characterise the space of actions under threat, investigate the cognitive mechanisms by which these actions are selected in different scenarios, and describe them with computational algorithms that allow quantitative predictions. To assess their systems-level neural implementation, we use wearable magnetoencephalography (MEG) while people move freely and interact with the virtual environment.
Threat forecasting
Psychotherapy for anxiety disorders is based on learning mechanisms. We seek to optimise psychotherapeutic interventions by better understanding the underlying learning dynamics. We use a computational approach to study how the neural system establishes and adjusts threat predictions. Experimentally, we combine simplified cross-species laboratory models of threat prediction (such as Pavlovian fear conditioning) with various behavioural and physiological assessments. We develop these assessments of implicit learning to achieve optimised signal-to-noise ratio. To verify the neural systems underlying our learning indices we capitalise on patients with rare subcortical lesions. Our novel data analysis methods are freely available to the community (PsPM – psychophysiological modelling).
Novel treatments
In order to facilitate translation into clinical practice, we are testing a range of candidate treatments in laboratory models of psychiatric disorders. Our focus is on interventions that reduce synaptic plasticity to impair the consolidation of threat predictions, or to disrupt established predictions. Our approach encompasses pharmacological interventions with synaptic plasticity-inhibiting drugs, temporary cortex lesions with transcranial magnetic stimulation guided by fMRI, and behavioural interventions.
Key Publications
Brookes, J., Hall, S., Frühholz, S., & Bach, D. R. (2023). Immersive VR for investigating threat avoidance: The VRthreat toolkit for Unity. Behavior Research Methods, 1-15. doi:10.3758/s13428-023-02241-y
Sporrer, J. K., Brookes, J., Hall, S., Zabbah, S., Hernandez, U. D. S., & Bach, D. R. (2023). Functional sophistication in human escape. iScience, 26 (11), 108240. doi:10.1016/j.isci.2023.108240
Ojala, K. E., Staib, M., Gerster, S., Ruff, C. C., & Bach, D. R. (2022). Inhibiting human aversive memory by transcranial theta-burst stimulation to the primary sensory cortex. Biological psychiatry, 92(2), 149-157. doi:10.1016/j.biopsych.2022.01.021
Bach, D. R. (2022). Cross-species anxiety tests in psychiatry: pitfalls and promises. Molecular Psychiatry, 27(1), 154-163. doi:10.1038/s41380-021-01299-4
Castegnetti, G., Tzovara, A., Khemka, S., Melinščak, F., Barnes, G. R., Dolan, R. J., & Bach, D. R. (2020). Representation of probabilistic outcomes during risky decision-making. Nature communications, 11(1), 2419. doi:10.1038/s41467-020-16202-y
Team
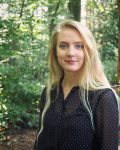
Juliana Sporrer
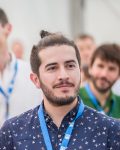
Ulises Daniel Serratos Hernández
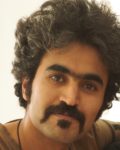
Sajjad Zabbah
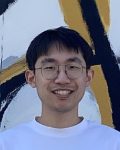
Huaiyu Liu
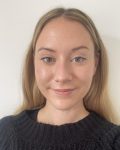
Josie Linnell
- View all publications by the Threat Avoidance team