Theoretical Neurobiology
Research team
We develop and apply advanced mathematical techniques that provide testable, theoretical models of dynamic brain function in health and disease.
We treat the brain as a (Bayesian) statistical organ that predicts current and future events based on beliefs about the state of the world. Perception and action operate under one unified (free energy) principle – and its corollary active inference – whereby we make hypotheses about the most likely cause of our observations and update these hypotheses to minimise uncertainty about the world.
We model brain function (e.g., vision, motor control, learning, decision making, speech recognition) using active inference, by associating neuronal dynamics with a gradient flow on variational free energy, in neurobiologically plausible architectures. Behavioural and neural responses are simulated under different conditions (e.g., prior beliefs, noise and optimization processes) and used to predict behavioural, neuronal or physiological observations (e.g., the P300 and mismatch negativity in EEG signals that can be used to measure Bayesian surprise). The models that best explain the data can then be selected (and validated) using Bayesian model comparison.
Neurobiologically plausible implementations of the models are tested and optimised, using a range of data from non-invasive neuroimaging (fMRI, EEG, MEG) and invasive electrode recordings. Neuronal architectures are inferred from neuroimaging data by model inversion. For example, we used EEG recordings to estimate receptor function in N-methyl-d-aspartate (NMDA) antibody encephalitis (Symmonds et al., 2018).
The software we use to analyse neuronal dynamics, Dynamic Causal Modelling has been used recently to:
(i) distinguish forward and backward neuronal signalling in different layers and levels of the cortex (Preller et al., 2019),
(ii) specify the mechanisms of neuronal communication at the synaptic and neurotransmitter level (Rosch et al., 2018)
(iii) elucidate the principles of inference in the brain and its role in psychopathology (Hamburg et al., 2019), and
(iv) detect abnormalities in neurovascular coupling (Friston et al., 2017b).
Recent developments to the underlying theoretical formulation include: deep (hierarchical) temporal models where the outcomes of one (slower) level generate the hidden states at a lower (faster) level (e.g. reading), mixed generative models where continuous sensations inform discrete representation (e.g. speech recognition), and continuous space models where continuous sensations inform continuous representations (e.g. motor control).
Clinically, we aim to understand pathophysiology and psychopathology in computational (i.e. functional) terms – in a variety of neurological and psychiatric syndromes.
Team
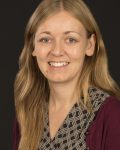
Jakub Limanowski
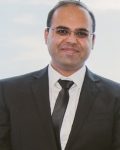
Amirhossein Jafarian
Andrew Levy
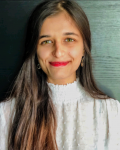
Noor Sajid
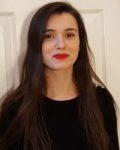
Victorita Neacsu
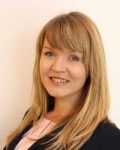
Edda Bilek
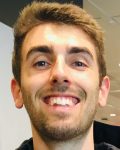
Lancelot Da Costa
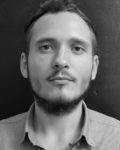
Sebastijan Veselic
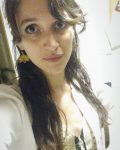
Laura Convertino
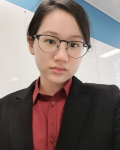